Topic: Data Science Application Climate Science
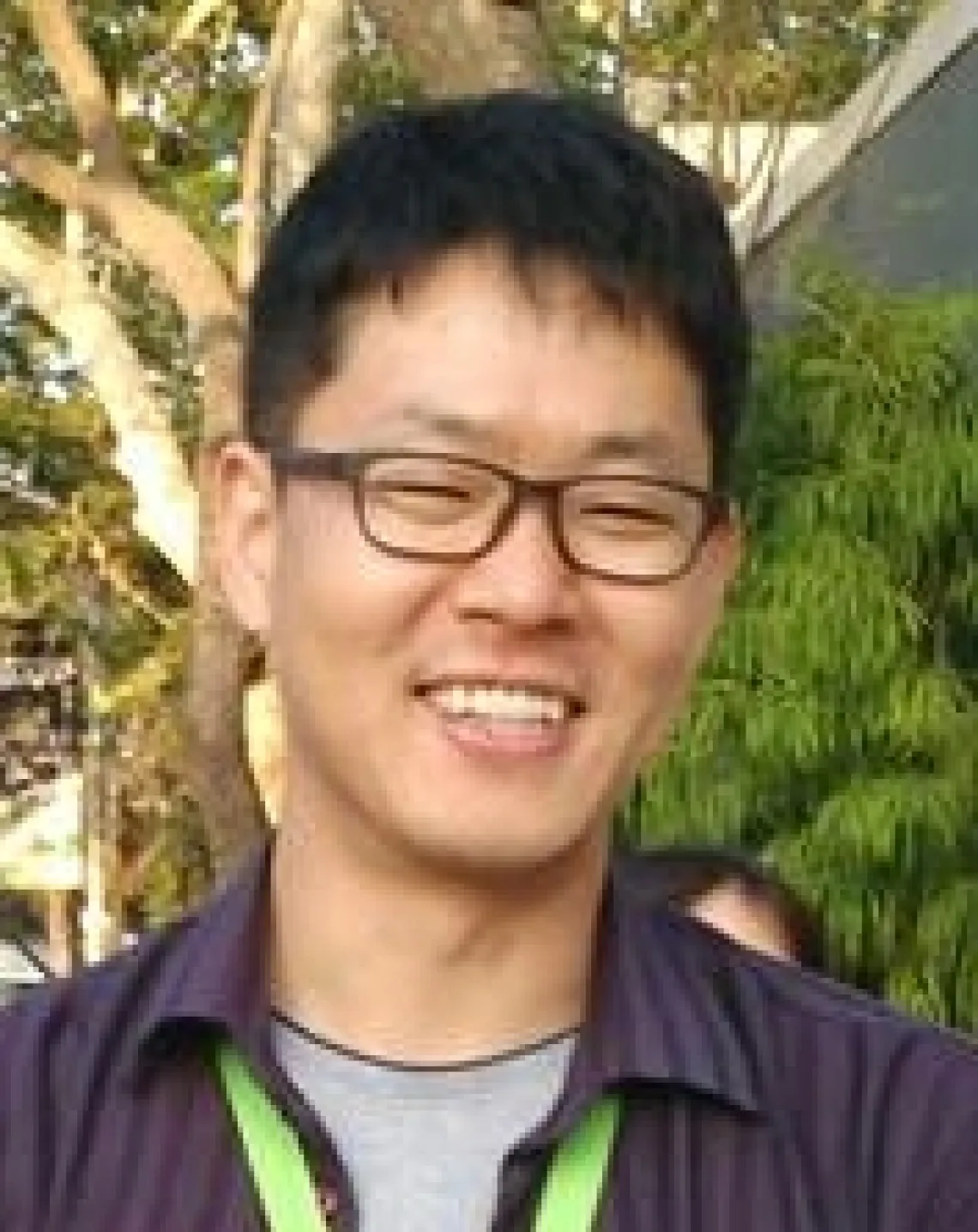
When
Where
Seminar Format
Available remotely via Zoom: Contact the department to subscribe to the email list (zoom link provided in announcement).
Abstract
Topology is the study of shapes. Topological data analysis (TDA) is an emerging machinery at the interface of algebraic topology, machine learning (ML), and statistics. TDA has shown a high utility in a diverse range of applications, from social studies to digital health care to power systems. While geometrical methods, such as TDA, continue to gain popularity in statistical sciences and ML, from causal inference to deep learning on manifolds, the utility of geometric methods for assessing the spatial characteristics of Earth science datasets is yet untapped. Topological information on the inherent data shape can provide invaluable insights into the latent data structure and organization and can serve a leading role in understanding spatiotemporal dynamic patterns of observations and climate models.
Bio
Dr. Hugo Kyo Lee from NASA's Jet Propulsion Laboratory (JPL) is an atmospheric scientist with a background in research and data analysis, specializing in climate science and remote sensing. Serving as the Principal Investigator of NASA's Advanced Information Systems and Technology (AIST) project and JPL's Regional Climate Model Evaluation System (RCMES), Dr. Lee has played a role in advancing our understanding of climate systems and their impact on the Earth's climate system at JPL for the last 11 years. Dr. Lee received a Ph.D. in Atmospheric Sciences from the University of Illinois at Urbana-Champaign in 2012 and a B.S. in Atmospheric Sciences from Seoul National University, Korea, in 2002.
Hugo Kyo Lee: [Email: Huikyo.Lee@jpl.nasa.gov | Jet Propulsion Laboratory | Google Scholar]