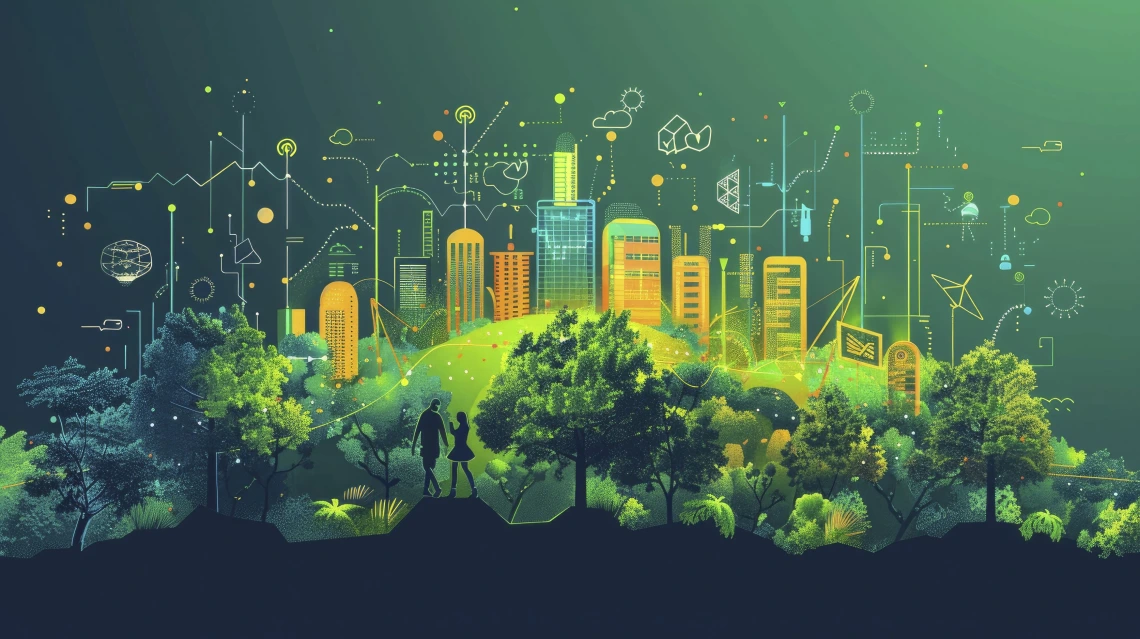
When
Weekly Seminar Format
Available in-person and via Zoom. Contact the department to subscribe to the email list (zoom link provided in announcement).
Abstract
In the Geosciences, we seek to develop knowledge about systems that are spatially extensive and that evolve dynamically over time. Meanwhile, Information Theory (IT) seeks to provide a rigorous formal basis for characterizing and quantifying “information” as provided by both data and models/theory, so that we can design and implement robust statistical procedures for inference and scientific hypothesis testing. And the rapidly developing field of Machine-Learning (ML) is providing deep insights into how we can construct representations that enable robust and rapid learning from data. This talk is about the synergy achievable by bringing together these complementary perspectives.
In this context, the “Goal of Science” is to discover a Representation (including Embedding) that is (a) Interpretable, and of (b) Minimal Description Length, that results in (c) Minimal Predictive Surprise, (d) Generalizes well when tested against reality (both spatially and temporally) and finally (e) supports Scientific Reasoning. We know that Shannon IT addresses predictive surprise and Algorithmic IT addresses description length (representational complexity) and to some extent the issue of generalization. I will argue that we need to develop a robust theory of “Representational Information” that addresses the issues of Interpretability, Generalizability and Support for Reasoning, and the role that representational choices play in aligning physical geoscientific knowledge/theory with knowledge provided by data. This requires an understanding of the role played by language in the construction of descriptions. I will attempt to illustrate these ideas with specific examples/results from the field of Hydrology.
Bio
Hoshin Gupta is Regents Professor of Hydrology and Atmospheric Sciences at The University of Arizona. He received his BS in Civil Engineering from IIT Bombay, and MS and PhD degrees in Systems Engineering from Case Western Reserve University. His broad interest is in how “Learning” and “Reasoning” happen through the development and use of “Models,” and more specifically in how to combine Physics-Based Knowledge with Machine Learning (via Information Theory) for developing Earth & Environmental Systems Models that can progressively learn from interactions with the environment. He is a Fellow of the American Geophysical Union and the American Meteorological Society, and he received AMS’s RE Horton Lecture Award in 2017 and EGU’s Dalton Medal in 2014.