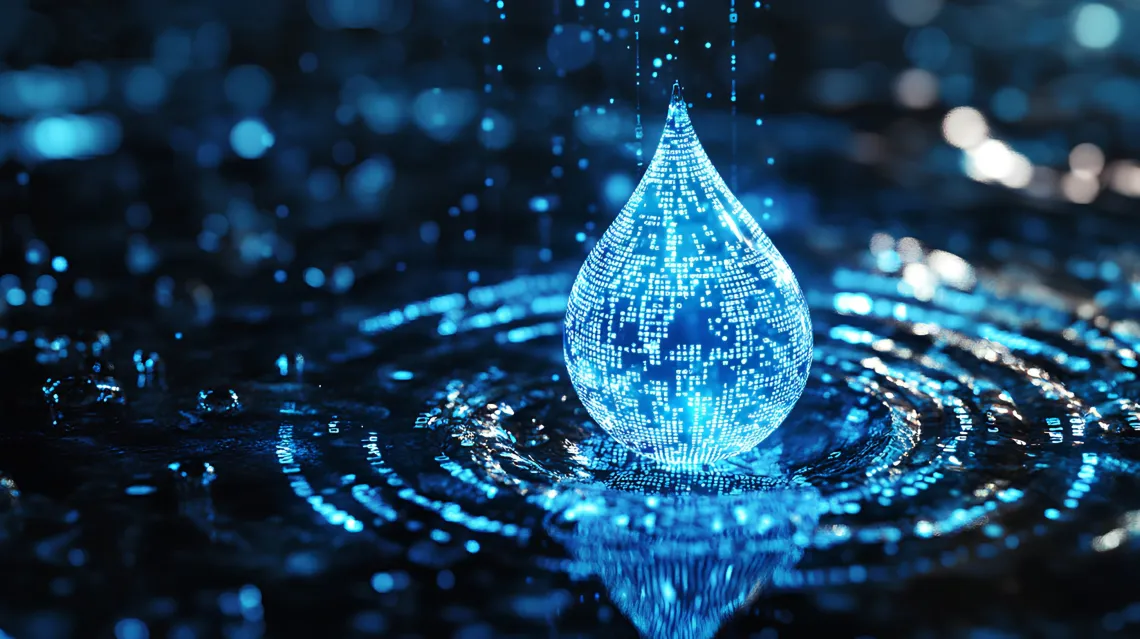
When
Weekly Seminar Format
Available in-person and via Zoom. Contact the department to subscribe to the email list (zoom link provided in announcement).
Abstract
Multi-scale hydrological and climatological phenomena, captured in geospatial data and large-domain simulations, can be exploited using machine learning (ML) to enhance predictions and process understanding across hydrological scales and boundaries. Large-domain hydrologic simulations are increasingly used for high-stakes forecasts. However, the potential of continental-scale hydrological data is not yet fully realized. ML is utilized to exploit large geospatial data and the full geospatial context of large-domain hydrological predictions, enhancing the accuracy of forecasts across diverse hydrological conditions, aiding in monitoring and response to natural hazards and improving our understanding of hydrological processes and global water cycle dynamics.
- Using the U.S. National Water Model and a convolutional neural network (NWM-CNN), we achieved better accuracy in flood predictions than the deployed HAND method, demonstrating the value of large-domain data with rich geospatial hydrological context (Frame et al., 2024).
- GOES imagery and a CNN were used to identify atmospheric rivers on the Pacific West Coast, highlighting the usefulness of spatial context data for real-time monitoring (Yadav et al., 2024).
- An ML model, utilizing GOES imagery, predicted snow water equivalent (SWE) at the catchment scale, showing a significant correlation with full-disc GOES images (Neisary et al., 2024).
- ML models can manage spatiotemporal biases effectively, providing a deeper understanding of hydrological processes beyond catchment boundaries (Frame et al., 2023).
Bio
Dr. Jonathan Frame: Google Scholar
Dr. Jonathan Frame is an Assistant Professor of Artificial Intelligence/Machine Learning in Geological Sciences at the University of Alabama and an Affiliate Faculty member of the Alabama Water Institute. He earned his PhD from the University of Alabama in 2022, following an MS in Civil Engineering from the University of California, Irvine, and a BS in Earth Systems Science from California State University, Monterey. Dr. Frame’s research focuses on integrating deep learning models with hydrological systems, particularly in flood prediction, runoff processes, and water resources management. He has published extensively on topics such as flood mapping using the U.S. National Water Model, machine learning integration in hydrologic models, and deep learning predictions of extreme weather events. His professional experience includes roles at Floodbase, NOAA’s National Water Center, and consulting work in water resources engineering, where he applied AI to improve flood prediction, water management, and environmental sustainability.